A soft robust model for optimization under ambiguity
Date
2010-07-01
Authors
Journal Title
Journal ISSN
Volume Title
Repository Usage Stats
views
downloads
Citation Stats
Abstract
In this paper, we propose a framework for robust optimization that relaxes the standard notion of robustness by allowing the decision maker to vary the protection level in a smooth way across the uncertainty set. We apply our approach to the problem of maximizing the expected value of a payoff function when the underlying distribution is ambiguous and therefore robustness is relevant. Our primary objective is to develop this framework and relate it to the standard notion of robustness, which deals with only a single guarantee across one uncertainty set. First, we show that our approach connects closely to the theory of convex risk measures. We show that the complexity of this approach is equivalent to that of solving a small number of standard robust problems. We then investigate the conservatism benefits and downside probability guarantees implied by this approach and compare to the standard robust approach. Finally, we illustrate theme thodology on an asset allocation example consisting of historical market data over a 25-year investment horizon and find in every case we explore that relaxing standard robustness with soft robustness yields a seemingly favorable risk-return trade-off: each case results in a higher out-of-sample expected return for a relatively minor degradation of out-of-sample downside performance. © 2010 INFORMS.
Type
Department
Description
Provenance
Subjects
Citation
Permalink
Published Version (Please cite this version)
Publication Info
Ben-Tal, A, D Bertsimas and DB Brown (2010). A soft robust model for optimization under ambiguity. Operations Research, 58(4 PART 2). pp. 1220–1234. 10.1287/opre.1100.0821 Retrieved from https://hdl.handle.net/10161/4438.
This is constructed from limited available data and may be imprecise. To cite this article, please review & use the official citation provided by the journal.
Collections
Scholars@Duke
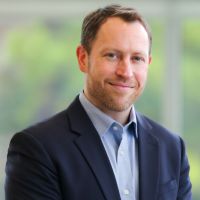
David B. Brown
David B. Brown is the Snow Family Business Professor in Decision Sciences and the Faculty Director for the Center for Energy, Development and Global Environment (EDGE) at Duke University's Fuqua School of Business.
Professor Brown's research focuses on designing and analyzing algorithms for decision problems involving uncertainty and complex tradeoffs. This work is methodological in nature and cuts across various application areas. Part of the GRACE project funded by the US Department of Energy's Advanced Research Projects Agency, Professor Brown is actively working with researchers at Duke and several other institutions on improving the efficiency and reliability of electricity grid operations in the face of uncertainty in renewable energy sources.
His recent research also includes developing and analyzing solution techniques for problems such as network revenue management, dynamic pricing in shared vehicle systems, stochastic scheduling problems, and sequential search problems. Professor Brown's research has appeared in publications such as Management Science and Operations Research, and the Institute for Operations Research and the Management Sciences (INFORMS) has recognized his research with several awards. At Fuqua, he has taught Decision Models, Data Analytics and Applications, Probability and Statistics, and Convex Optimization, and he has won teaching awards in multiple programs.
Professor Brown received a Bachelor's and Master's of Science in Electrical Engineering from Stanford University and has been on the faculty at Fuqua since receiving his Ph.D. in Electrical Engineering and Computer Science from MIT.
Unless otherwise indicated, scholarly articles published by Duke faculty members are made available here with a CC-BY-NC (Creative Commons Attribution Non-Commercial) license, as enabled by the Duke Open Access Policy. If you wish to use the materials in ways not already permitted under CC-BY-NC, please consult the copyright owner. Other materials are made available here through the author’s grant of a non-exclusive license to make their work openly accessible.