Evaluating functional network inference using simulations of complex biological systems.
Abstract
MOTIVATION: Although many network inference algorithms have been presented in the bioinformatics literature, no suitable approach has been formulated for evaluating their effectiveness at recovering models of complex biological systems from limited data. To overcome this limitation, we propose an approach to evaluate network inference algorithms according to their ability to recover a complex functional network from biologically reasonable simulated data. RESULTS: We designed a simulator to generate data representing a complex biological system at multiple levels of organization: behaviour, neural anatomy, brain electrophysiology, and gene expression of songbirds. About 90% of the simulated variables are unregulated by other variables in the system and are included simply as distracters. We sampled the simulated data at intervals as one would sample from a biological system in practice, and then used the sampled data to evaluate the effectiveness of an algorithm we developed for functional network inference. We found that our algorithm is highly effective at recovering the functional network structure of the simulated system-including the irrelevance of unregulated variables-from sampled data alone. To assess the reproducibility of these results, we tested our inference algorithm on 50 separately simulated sets of data and it consistently recovered almost perfectly the complex functional network structure underlying the simulated data. To our knowledge, this is the first approach for evaluating the effectiveness of functional network inference algorithms at recovering models from limited data. Our simulation approach also enables researchers a priori to design experiments and data-collection protocols that are amenable to functional network inference.
Type
Department
Description
Provenance
Subjects
Citation
Permalink
Collections
Scholars@Duke

Erich David Jarvis
Dr. Jarvis' laboratory studies the neurobiology of vocal communication. Emphasis is placed on the molecular pathways involved in the perception and production of learned vocalizations. They use an integrative approach that combines behavioral, anatomical, electrophysiological and molecular biological techniques. The main animal model used is songbirds, one of the few vertebrate groups that evolved the ability to learn vocalizations. The generality of the discoveries is tested in other vocal learning orders, such as parrots and hummingbirds, as well as non-vocal learners, such as pigeons and non-human primates. Some of the questions require performing behavior/molecular biology experiments in freely ranging animals, such as hummingbirds in tropical forest of Brazil. Recent results show that in songbirds, parrots and hummingbirds, perception and production of song are accompanied by anatomically distinct patterns of gene expression. All three groups were found to exhibit vocally-activated gene expression in exactly 7 forebrain nuclei that are very similar to each other. These structures for vocal learning and production are thought to have evolved independently within the past 70 million years, since they are absent from interrelated non-vocal learning orders. One structure, Area X of the basal ganglia's striatum in songbirds, shows large differential gene activation depending on the social context in which the bird sings. These differences may reflect a semantic content of song, perhaps similar to human language.
The overall goal of the research is to advance knowledge of the neural mechanisms for vocal learning and basic mechanisms of brain function. These goals are further achieved by combined collaborative efforts with the laboratories of Drs. Mooney and Nowicki at Duke University, who study respectively behavior and electrophysiological aspects of songbird vocal communication.
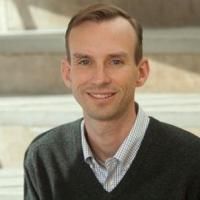
Alexander J. Hartemink
Computational biology, machine learning, Bayesian statistics, transcriptional regulation, genomics and epigenomics, graphical models, Bayesian networks, hidden Markov models, systems biology, computational neurobiology, classification, feature selection
Unless otherwise indicated, scholarly articles published by Duke faculty members are made available here with a CC-BY-NC (Creative Commons Attribution Non-Commercial) license, as enabled by the Duke Open Access Policy. If you wish to use the materials in ways not already permitted under CC-BY-NC, please consult the copyright owner. Other materials are made available here through the author’s grant of a non-exclusive license to make their work openly accessible.