Derivation of a Risk Assessment Tool for Prediction of Long-Term Pain Intensity Reduction After Physical Therapy.
Date
2021-01
Journal Title
Journal ISSN
Volume Title
Repository Usage Stats
views
downloads
Citation Stats
Attention Stats
Abstract
Rationale
Risk assessment tools can improve clinical decision-making for individuals with musculoskeletal pain, but do not currently exist for predicting reduction of pain intensity as an outcome from physical therapy.Aims and objective
The objective of this study was to develop a tool that predicts failure to achieve a 50% pain intensity reduction by 1) determining the appropriate statistical model to inform the tool and 2) select the model that considers the tradeoff between clinical feasibility and statistical accuracy.Methods
This was a retrospective, secondary data analysis of the Optimal Screening for Prediction of Referral and Outcome (OSPRO) cohort. Two hundred and seventy-nine individuals seeking physical therapy for neck, shoulder, back, or knee pain who completed 12-month follow-up were included. Two modeling approaches were taken: a longitudinal model included demographics, presence of previous episodes of pain, and regions of pain in addition to baseline and change in OSPRO Yellow Flag scores to 12 months; two comparison models included the same predictors but assessed only baseline and early change (4 weeks) scores. The primary outcome was failure to achieve a 50% reduction in pain intensity score at 12 months. We compared the area under the curve (AUC) to assess the performance of each candidate model and to determine which to inform the Personalized Pain Prediction (P3) risk assessment tool.Results
The baseline only and early change models demonstrated lower accuracy (AUC=0.68 and 0.71, respectively) than the longitudinal model (0.79) but were within an acceptable predictive range. Therefore, both baseline and early change models were used to inform the P3 risk assessment tool.Conclusion
The P3 tool provides physical therapists with a data-driven approach to identify patients who may be at risk for not achieving improvements in pain intensity following physical therapy.Type
Department
Description
Provenance
Citation
Permalink
Published Version (Please cite this version)
Publication Info
Horn, Maggie E, Steven Z George, Cai Li, Sheng Luo and Trevor A Lentz (2021). Derivation of a Risk Assessment Tool for Prediction of Long-Term Pain Intensity Reduction After Physical Therapy. Journal of pain research, 14. pp. 1515–1524. 10.2147/jpr.s305973 Retrieved from https://hdl.handle.net/10161/23410.
This is constructed from limited available data and may be imprecise. To cite this article, please review & use the official citation provided by the journal.
Collections
Scholars@Duke

Maggie Elizabeth Horn

Steven Zachary George
Dr. George’s primary interest is research involving biopsychosocial models for the prevention and treatment of chronic musculoskeletal pain disorders. His long term goals are to 1) improve accuracy for predicting who is going to develop chronic pain; and 2) identify non-pharmacological treatment options that limit the development of chronic pain conditions. Dr. George is an active member of the American Physical Therapy Association, United States Association of the Study of Pain, and International Association for the Study of Pain.
Dr. George’s research projects have been supported by the National Institutes of Health, Department of Defense, and Orthopaedic Academy of the American Physical Therapy Association. Dr. George and his collaborators have authored over 330 peer-reviewed publications in leading medical, orthopaedic surgery, physical therapy, rehabilitation, and pain research journals. He currently serves as Editor-in-Chief for the Physical Therapy & Rehabilitation Journal. Dr. George has also been involved with clinical practice guideline development for the Academy of Orthopaedic Physical Therapy and the American Psychological Association.
Dr. George has been recognized with prestigious research awards from the American Physical Therapy Association, American Pain Society, and International Association for the Study of Pain. For example from the American Physical Therapy Association: he was named the 21st John H.P. Maley Lecturer, recognized as a Catherine Worthingham Fellow in 2017, and selected for the Marian Williams Award for Research in Physical Therapy in 2022.
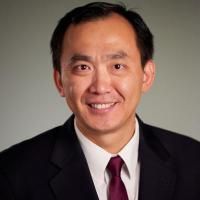
Sheng Luo
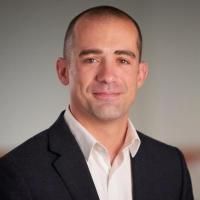
Trevor A. Lentz
Unless otherwise indicated, scholarly articles published by Duke faculty members are made available here with a CC-BY-NC (Creative Commons Attribution Non-Commercial) license, as enabled by the Duke Open Access Policy. If you wish to use the materials in ways not already permitted under CC-BY-NC, please consult the copyright owner. Other materials are made available here through the author’s grant of a non-exclusive license to make their work openly accessible.