Detection of Lung Nodules in Micro-CT Imaging Using Deep Learning
Abstract
<jats:p>We are developing imaging methods for a co-clinical trial investigating synergy between immunotherapy and radiotherapy. We perform longitudinal micro-computed tomography (micro-CT) of mice to detect lung metastasis after treatment. This work explores deep learning (DL) as a fast approach for automated lung nodule detection. We used data from control mice both with and without primary lung tumors. To augment the number of training sets, we have simulated data using real augmented tumors inserted into micro-CT scans. We employed a convolutional neural network (CNN), trained with four competing types of training data: (1) simulated only, (2) real only, (3) simulated and real, and (4) pretraining on simulated followed with real data. We evaluated our model performance using precision and recall curves, as well as receiver operating curves (ROC) and their area under the curve (AUC). The AUC appears to be almost identical (0.76–0.77) for all four cases. However, the combination of real and synthetic data was shown to improve precision by 8%. Smaller tumors have lower rates of detection than larger ones, with networks trained on real data showing better performance. Our work suggests that DL is a promising approach for fast and relatively accurate detection of lung tumors in mice.</jats:p>
Type
Department
Description
Provenance
Subjects
Citation
Permalink
Published Version (Please cite this version)
Publication Info
Holbrook, Matthew D, Darin P Clark, Rutulkumar Patel, Yi Qi, Alex M Bassil, Yvonne M Mowery and Cristian T Badea (n.d.). Detection of Lung Nodules in Micro-CT Imaging Using Deep Learning. Tomography, 7(3). pp. 358–372. 10.3390/tomography7030032 Retrieved from https://hdl.handle.net/10161/23575.
This is constructed from limited available data and may be imprecise. To cite this article, please review & use the official citation provided by the journal.
Collections
Scholars@Duke
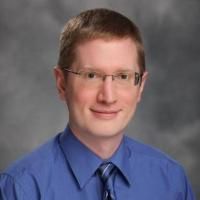
Darin Clark
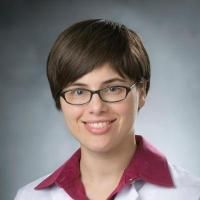
Yvonne Marie Mowery
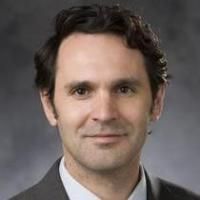
Cristian Tudorel Badea
- Our lab's research focus lies primarily in developing novel quantitative imaging systems, reconstruction algorithms and analysis methods. My major expertise is in preclinical CT.
- Currently, we are particularly interested in developing novel strategies for spectral CT imaging using nanoparticle-based contrast agents for theranostics (i.e. therapy and diagnostics).
- We are also engaged in developing new approaches for multidimensional CT image reconstruction suitable to address difficult undersampling cases in cardiac and spectral CT (dual energy and photon counting) using compressed sensing and/or deep learning.
- We are involved in co-clinical cancer trials and I serve as the Principal Investigator on the U24 Duke Preclinical Research Resources for Quantitative Imaging Biomarkers part of the NCI Co-Clinical Imaging Research Resources Program network (CIRP).
Unless otherwise indicated, scholarly articles published by Duke faculty members are made available here with a CC-BY-NC (Creative Commons Attribution Non-Commercial) license, as enabled by the Duke Open Access Policy. If you wish to use the materials in ways not already permitted under CC-BY-NC, please consult the copyright owner. Other materials are made available here through the author’s grant of a non-exclusive license to make their work openly accessible.