Functional survival forests for multivariate longitudinal outcomes: Dynamic prediction of Alzheimer's disease progression.
Date
2020-07-29
Authors
Journal Title
Journal ISSN
Volume Title
Repository Usage Stats
views
downloads
Citation Stats
Abstract
The random survival forest (RSF) is a non-parametric alternative to the Cox proportional hazards model in modeling time-to-event data. In this article, we developed a modeling framework to incorporate multivariate longitudinal data in the model building process to enhance the predictive performance of RSF. To extract the essential features of the multivariate longitudinal outcomes, two methods were adopted and compared: multivariate functional principal component analysis and multivariate fast covariance estimation for sparse functional data. These resulting features, which capture the trajectories of the multiple longitudinal outcomes, are then included as time-independent predictors in the subsequent RSF model. This non-parametric modeling framework, denoted as functional survival forests, is better at capturing the various trends in both the longitudinal outcomes and the survival model which may be difficult to model using only parametric approaches. These advantages are demonstrated through simulations and applications to the Alzheimer's Disease Neuroimaging Initiative.
Type
Department
Description
Provenance
Citation
Permalink
Published Version (Please cite this version)
Publication Info
Lin, Jeffrey, Kan Li and Sheng Luo (2020). Functional survival forests for multivariate longitudinal outcomes: Dynamic prediction of Alzheimer's disease progression. Statistical methods in medical research. p. 962280220941532. 10.1177/0962280220941532 Retrieved from https://hdl.handle.net/10161/21389.
This is constructed from limited available data and may be imprecise. To cite this article, please review & use the official citation provided by the journal.
Collections
Scholars@Duke
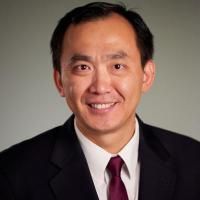
Sheng Luo
Unless otherwise indicated, scholarly articles published by Duke faculty members are made available here with a CC-BY-NC (Creative Commons Attribution Non-Commercial) license, as enabled by the Duke Open Access Policy. If you wish to use the materials in ways not already permitted under CC-BY-NC, please consult the copyright owner. Other materials are made available here through the author’s grant of a non-exclusive license to make their work openly accessible.