Artificial Intelligence Based Hierarchical Clustering of Patient Types and Intervention Categories in Adult Spinal Deformity Surgery: Towards a New Classification Scheme that Predicts Quality and Value.
Date
2019-07
Journal Title
Journal ISSN
Volume Title
Repository Usage Stats
views
downloads
Citation Stats
Abstract
STUDY DESIGN:Retrospective review of prospectively-collected, multicenter adult spinal deformity (ASD) databases. OBJECTIVE:To apply artificial intelligence (AI)-based hierarchical clustering as a step toward a classification scheme that optimizes overall quality, value, and safety for ASD surgery. SUMMARY OF BACKGROUND DATA:Prior ASD classifications have focused on radiographic parameters associated with patient reported outcomes. Recent work suggests there are many other impactful preoperative data points. However, the ability to segregate patient patterns manually based on hundreds of data points is beyond practical application for surgeons. Unsupervised machine-based clustering of patient types alongside surgical options may simplify analysis of ASD patient types, procedures, and outcomes. METHODS:Two prospective cohorts were queried for surgical ASD patients with baseline, 1-year, and 2-year SRS-22/Oswestry Disability Index/SF-36v2 data. Two dendrograms were fitted, one with surgical features and one with patient characteristics. Both were built with Ward distances and optimized with the gap method. For each possible n patient cluster by m surgery, normalized 2-year improvement and major complication rates were computed. RESULTS:Five hundred-seventy patients were included. Three optimal patient types were identified: young with coronal plane deformity (YC, n = 195), older with prior spine surgeries (ORev, n = 157), and older without prior spine surgeries (OPrim, n = 218). Osteotomy type, instrumentation and interbody fusion were combined to define four surgical clusters. The intersection of patient-based and surgery-based clusters yielded 12 subgroups, with major complication rates ranging from 0% to 51.8% and 2-year normalized improvement ranging from -0.1% for SF36v2 MCS in cluster [1,3] to 100.2% for SRS self-image score in cluster [2,1]. CONCLUSION:Unsupervised hierarchical clustering can identify data patterns that may augment preoperative decision-making through construction of a 2-year risk-benefit grid. In addition to creating a novel AI-based ASD classification, pattern identification may facilitate treatment optimization by educating surgeons on which treatment patterns yield optimal improvement with lowest risk. LEVEL OF EVIDENCE:4.
Type
Department
Description
Provenance
Citation
Permalink
Published Version (Please cite this version)
Publication Info
Ames, Christopher P, Justin S Smith, Ferran Pellisé, Michael Kelly, Ahmet Alanay, Emre Acaroğlu, Francisco Javier Sánchez Pérez-Grueso, Frank Kleinstück, et al. (2019). Artificial Intelligence Based Hierarchical Clustering of Patient Types and Intervention Categories in Adult Spinal Deformity Surgery: Towards a New Classification Scheme that Predicts Quality and Value. Spine, 44(13). pp. 915–926. 10.1097/brs.0000000000002974 Retrieved from https://hdl.handle.net/10161/19126.
This is constructed from limited available data and may be imprecise. To cite this article, please review & use the official citation provided by the journal.
Collections
Scholars@Duke
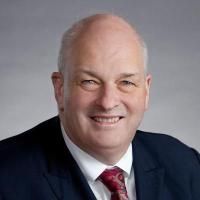
Christopher Ignatius Shaffrey
I have more than 25 years of experience treating patients of all ages with spinal disorders. I have had an interest in the management of spinal disorders since starting my medical education. I performed residencies in both orthopaedic surgery and neurosurgery to gain a comprehensive understanding of the entire range of spinal disorders. My goal has been to find innovative ways to manage the range of spinal conditions, straightforward to complex. I have a focus on managing patients with complex spinal disorders. My patient evaluation and management philosophy is to provide engaged, compassionate care that focuses on providing the simplest and least aggressive treatment option for a particular condition. In many cases, non-operative treatment options exist to improve a patient’s symptoms. I have been actively engaged in clinical research to find the best ways to manage spinal disorders in order to achieve better results with fewer complications.
Unless otherwise indicated, scholarly articles published by Duke faculty members are made available here with a CC-BY-NC (Creative Commons Attribution Non-Commercial) license, as enabled by the Duke Open Access Policy. If you wish to use the materials in ways not already permitted under CC-BY-NC, please consult the copyright owner. Other materials are made available here through the author’s grant of a non-exclusive license to make their work openly accessible.