The evolutionary forest algorithm.
Date
2007-08
Journal Title
Journal ISSN
Volume Title
Repository Usage Stats
views
downloads
Citation Stats
Abstract
Motivation
Gene genealogies offer a powerful context for inferences about the evolutionary process based on presently segregating DNA variation. In many cases, it is the distribution of population parameters, marginalized over the effectively infinite-dimensional tree space, that is of interest. Our evolutionary forest (EF) algorithm uses Monte Carlo methods to generate posterior distributions of population parameters. A novel feature is the updating of parameter values based on a probability measure defined on an ensemble of histories (a forest of genealogies), rather than a single tree.Results
The EF algorithm generates samples from the correct marginal distribution of population parameters. Applied to actual data from closely related fruit fly species, it rapidly converged to posterior distributions that closely approximated the exact posteriors generated through massive computational effort. Applied to simulated data, it generated credible intervals that covered the actual parameter values in accordance with the nominal probabilities.Availability
A C++ implementation of this method is freely accessible at http://www.isds.duke.edu/~scl13Type
Department
Description
Provenance
Citation
Permalink
Published Version (Please cite this version)
Publication Info
Leman, Scotland C, Marcy K Uyenoyama, Michael Lavine and Yuguo Chen (2007). The evolutionary forest algorithm. Bioinformatics (Oxford, England), 23(15). pp. 1962–1968. 10.1093/bioinformatics/btm264 Retrieved from https://hdl.handle.net/10161/25954.
This is constructed from limited available data and may be imprecise. To cite this article, please review & use the official citation provided by the journal.
Collections
Scholars@Duke
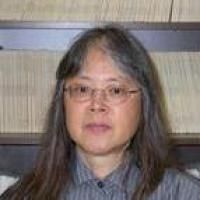
Marcy K. Uyenoyama
Marcy Uyenoyama studies mechanisms of evolutionary change at the molecular and population levels. Among the questions under study include the prediction and detection of the effects of natural selection on genomic structure. A major area of research addresses the development of maximum-likelihood and Bayesian methods for inferring evolutionary processes from the pattern of molecular variation. Evolutionary processes currently under study include characterization of population structure across genomes.
Unless otherwise indicated, scholarly articles published by Duke faculty members are made available here with a CC-BY-NC (Creative Commons Attribution Non-Commercial) license, as enabled by the Duke Open Access Policy. If you wish to use the materials in ways not already permitted under CC-BY-NC, please consult the copyright owner. Other materials are made available here through the author’s grant of a non-exclusive license to make their work openly accessible.