Data clustering based on Langevin annealing with a self-consistent potential
Date
2018-10-11
Journal Title
Journal ISSN
Volume Title
Repository Usage Stats
views
downloads
Citation Stats
Abstract
Type
Department
Description
Provenance
Subjects
Citation
Permalink
Published Version (Please cite this version)
Publication Info
Lafata, K, Z Zhou, JG Liu and FF Yin (2018). Data clustering based on Langevin annealing with a self-consistent potential. Quarterly of Applied Mathematics, 77(3). pp. 591–613. 10.1090/qam/1521 Retrieved from https://hdl.handle.net/10161/19225.
This is constructed from limited available data and may be imprecise. To cite this article, please review & use the official citation provided by the journal.
Collections
Scholars@Duke

Kyle Jon Lafata
Kyle Lafata is the Thaddeus V. Samulski Associate Professor at Duke University with faculty appointments in Radiation Oncology, Radiology, Pathology, Medical Physics, and Electrical & Computer Engineering. He joined the faculty at Duke in 2020 following postdoctoral training at the US Department of Veterans Affairs. His dissertation work focused on the applied analysis of stochastic partial differential equations and high-dimensional image phenotyping, where he developed physics-based computational methods and soft-computing paradigms to interrogate images. These included stochastic modeling, self-organization, and quantum machine learning (i.e., an emerging branch of research that explores the methodological and structural similarities between quantum systems and learning systems).
Prof. Lafata has worked in various areas of computational medicine and biology, resulting in over 55 academic papers, 20 invited talks, and more than 60 national conference presentations. At Duke, the Lafata Laboratory focuses on the theory, development, and application of computational oncology. The lab interrogates disease at different length-scales of its biological organization via high-performance computing, multiscale modeling, advanced imaging technology, and the applied analysis of stochastic partial differential equations. Current research interests include tumor topology, cellular dynamics, tumor immune microenvironment, drivers of radiation resistance and immune dysregulation, molecular insight into tissue heterogeneity, and biologically-guided adaptative treatment strategies.
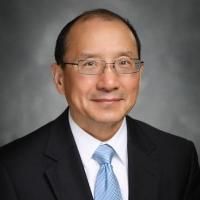
Fang-Fang Yin
Stereotactic radiosurgery, Stereotactic body radiation therapy, treatment planning optimization, knowledge guided radiation therapy, intensity-modulated radiation therapy, image-guided radiation therapy, oncological imaging and informatics
Unless otherwise indicated, scholarly articles published by Duke faculty members are made available here with a CC-BY-NC (Creative Commons Attribution Non-Commercial) license, as enabled by the Duke Open Access Policy. If you wish to use the materials in ways not already permitted under CC-BY-NC, please consult the copyright owner. Other materials are made available here through the author’s grant of a non-exclusive license to make their work openly accessible.