Universal Digital High Resolution Melt for the detection of pulmonary mold infections.
Date
2023-11-09
Journal Title
Journal ISSN
Volume Title
Repository Usage Stats
views
downloads
Citation Stats
Abstract
BACKGROUND: Invasive mold infections (IMIs) such as aspergillosis, mucormycosis, fusariosis, and lomentosporiosis are associated with high morbidity and mortality, particularly in immunocompromised patients, with mortality rates as high as 40% to 80%. Outcomes could be substantially improved with early initiation of appropriate antifungal therapy, yet early diagnosis remains difficult to establish and often requires multidisciplinary teams evaluating clinical and radiological findings plus supportive mycological findings. Universal digital high resolution melting analysis (U-dHRM) may enable rapid and robust diagnosis of IMI. This technology aims to accomplish timely pathogen detection at the single genome level by conducting broad-based amplification of microbial barcoding genes in a digital polymerase chain reaction (dPCR) format, followed by high-resolution melting of the DNA amplicons in each digital reaction to generate organism-specific melt curve signatures that are identified by machine learning. METHODS: A universal fungal assay was developed for U-dHRM and used to generate a database of melt curve signatures for 19 clinically relevant fungal pathogens. A machine learning algorithm (ML) was trained to automatically classify these 19 fungal melt curves and detect novel melt curves. Performance was assessed on 73 clinical bronchoalveolar lavage (BAL) samples from patients suspected of IMI. Novel curves were identified by micropipetting U-dHRM reactions and Sanger sequencing amplicons. RESULTS: U-dHRM achieved an average of 97% fungal organism identification accuracy and a turn-around-time of 4hrs. Pathogenic molds (Aspergillus, Mucorales, Lomentospora and Fusarium) were detected by U-dHRM in 73% of BALF samples suspected of IMI. Mixtures of pathogenic molds were detected in 19%. U-dHRM demonstrated good sensitivity for IMI, as defined by current diagnostic criteria, when clinical findings were also considered. CONCLUSIONS: U-dHRM showed promising performance as a separate or combination diagnostic approach to standard mycological tests. The speed of U-dHRM and its ability to simultaneously identify and quantify clinically relevant mold pathogens in polymicrobial samples as well as detect emerging opportunistic pathogens may provide information that could aid in treatment decisions and improve patient outcomes.
Type
Department
Description
Provenance
Subjects
Citation
Permalink
Published Version (Please cite this version)
Publication Info
Goshia, Tyler, April Aralar, Nathan Wiederhold, Jeffrey D Jenks, Sanjay R Mehta, Mridu Sinha, Aprajita Karmakar, Ankit Sharma, et al. (2023). Universal Digital High Resolution Melt for the detection of pulmonary mold infections. bioRxiv. 10.1101/2023.11.09.566457 Retrieved from https://hdl.handle.net/10161/29519.
This is constructed from limited available data and may be imprecise. To cite this article, please review & use the official citation provided by the journal.
Collections
Scholars@Duke
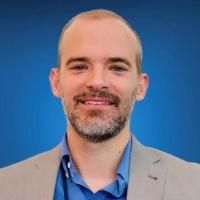
Jeffrey Daniel Jenks
Unless otherwise indicated, scholarly articles published by Duke faculty members are made available here with a CC-BY-NC (Creative Commons Attribution Non-Commercial) license, as enabled by the Duke Open Access Policy. If you wish to use the materials in ways not already permitted under CC-BY-NC, please consult the copyright owner. Other materials are made available here through the author’s grant of a non-exclusive license to make their work openly accessible.