Deep learning for the dynamic prediction of multivariate longitudinal and survival data.
Date
2022-03-28
Authors
Journal Title
Journal ISSN
Volume Title
Repository Usage Stats
views
downloads
Citation Stats
Abstract
The joint model for longitudinal and survival data improves time-to-event predictions by including longitudinal outcome variables in addition to baseline covariates. However, in practice, joint models may be limited by parametric assumptions in both the longitudinal and survival submodels. In addition, computational difficulties arise when considering multiple longitudinal outcomes due to the large number of random effects to be integrated out in the full likelihood. In this article, we discuss several recent machine learning methods for incorporating multivariate longitudinal data for time-to-event prediction. The presented methods use functional data analysis or convolutional neural networks to model the longitudinal data, both of which scale well to multiple longitudinal outcomes. In addition, we propose a novel architecture based on the transformer neural network, named TransformerJM, which jointly models longitudinal and time-to-event data. The prognostic abilities of each model are assessed and compared through both simulation and real data analysis on Alzheimer's disease datasets. Specifically, the models were evaluated based on their ability to dynamically update predictions as new longitudinal data becomes available. We showed that TransformerJM improves upon the predictive performance of existing methods across different scenarios.
Type
Department
Description
Provenance
Citation
Permalink
Published Version (Please cite this version)
Publication Info
Lin, Jeffrey, and Sheng Luo (2022). Deep learning for the dynamic prediction of multivariate longitudinal and survival data. Statistics in medicine. 10.1002/sim.9392 Retrieved from https://hdl.handle.net/10161/25067.
This is constructed from limited available data and may be imprecise. To cite this article, please review & use the official citation provided by the journal.
Collections
Scholars@Duke
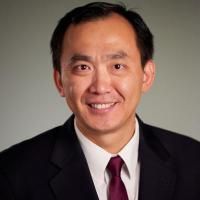
Sheng Luo
Unless otherwise indicated, scholarly articles published by Duke faculty members are made available here with a CC-BY-NC (Creative Commons Attribution Non-Commercial) license, as enabled by the Duke Open Access Policy. If you wish to use the materials in ways not already permitted under CC-BY-NC, please consult the copyright owner. Other materials are made available here through the author’s grant of a non-exclusive license to make their work openly accessible.