Bayesian inference of the number of factors in gene-expression analysis: application to human virus challenge studies
Date
2010-11-09
Journal Title
Journal ISSN
Volume Title
Repository Usage Stats
views
downloads
Citation Stats
Abstract
Type
Department
Description
Provenance
Subjects
Citation
Permalink
Published Version (Please cite this version)
Publication Info
Chen, Bo, Minhua Chen, John Paisley, Aimee Zaas, Christopher Woods, Geoffrey S Ginsburg, Alfred Hero, Joseph Lucas, et al. (2010). Bayesian inference of the number of factors in gene-expression analysis: application to human virus challenge studies. BMC BIOINFORMATICS, 11. p. 552. 10.1186/1471-2105-11-552 Retrieved from https://hdl.handle.net/10161/4336.
This is constructed from limited available data and may be imprecise. To cite this article, please review & use the official citation provided by the journal.
Collections
Scholars@Duke

Aimee Kirsch Zaas
Medical education
Genomic applications for diagnosis of infectious diseases
Genomic applications for prediction of infectious diseases

Christopher Wildrick Woods
1. Emerging Infections
2. Global Health
3. Epidemiology of infectious diseases
4. Clinical microbiology and diagnostics
5. Bioterrorism Preparedness
6. Surveillance for communicable diseases
7. Antimicrobial resistance

Geoffrey Steven Ginsburg
Dr. Geoffrey S. Ginsburg's research interests are in the development of novel paradigms for developing and translating genomic information into medical practice and the integration of personalized medicine into health care.
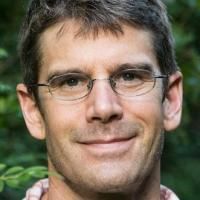
David B. Dunson
My research focuses on developing new tools for probabilistic learning from complex data - methods development is directly motivated by challenging applications in ecology/biodiversity, neuroscience, environmental health, criminal justice/fairness, and more. We seek to develop new modeling frameworks, algorithms and corresponding code that can be used routinely by scientists and decision makers. We are also interested in new inference framework and in studying theoretical properties of methods we develop.
Some highlight application areas:
(1) Modeling of biological communities and biodiversity - we are considering global data on fungi, insects, birds and animals including DNA sequences, images, audio, etc. Data contain large numbers of species unknown to science and we would like to learn about these new species, community network structure, and the impact of environmental change and climate.
(2) Brain connectomics - based on high resolution imaging data of the human brain, we are seeking to developing new statistical and machine learning models for relating brain networks to human traits and diseases.
(3) Environmental health & mixtures - we are building tools for relating chemical and other exposures (air pollution etc) to human health outcomes, accounting for spatial dependence in both exposures and disease. This includes an emphasis on infectious disease modeling, such as COVID-19.
Some statistical areas that play a prominent role in our methods development include models for low-dimensional structure in data (latent factors, clustering, geometric and manifold learning), flexible/nonparametric models (neural networks, Gaussian/spatial processes, other stochastic processes), Bayesian inference frameworks, efficient sampling and analytic approximation algorithms, and models for "object data" (trees, networks, images, spatial processes, etc).

Lawrence Carin
Lawrence Carin earned the BS, MS, and PhD degrees in electrical engineering at the University of Maryland, College Park, in 1985, 1986, and 1989, respectively. In 1989 he joined the Electrical Engineering Department at Brooklyn Polytechnic Institute (now part of NYU) as an Assistant Professor, and became an Associate Professor there in 1994. In September 1995 he joined the Electrical and Computer Engineering (ECE) Department at Duke University, where he is now a Professor. He was ECE Department Chair from 2011-2014, and Vice Provost and Vice President for Research from 2014-2020. He was the Provost at King Abdullah University of Science & Technology (KAUST) from 2020-2023, returning to Duke in 2023. From 2003-2014 he held the William H. Younger Distinguished Professorship, and since 2018 he has held the James L. Meriam Distinguished Professorship. Dr. Carin's research focuses on machine learning (ML) and artificial intelligence (AI). He publishes widely in the main ML/AI forums, and has addressed many applications of AI, including in medicine and security. He was co-founder of the small business Signal Innovations Group, which was acquired by BAE Systems in 2014, and in 2017 he co-founded the company Infinia ML, which was acquired by Aspirion in 2023. He is an IEEE Fellow.
Unless otherwise indicated, scholarly articles published by Duke faculty members are made available here with a CC-BY-NC (Creative Commons Attribution Non-Commercial) license, as enabled by the Duke Open Access Policy. If you wish to use the materials in ways not already permitted under CC-BY-NC, please consult the copyright owner. Other materials are made available here through the author’s grant of a non-exclusive license to make their work openly accessible.