Neural Network Approximation of Refinable Functions.
Abstract
In the desire to quantify the success of neural networks in deep learning and other applications, there is a great interest in understanding which functions are efficiently approximated by the outputs of neural networks. By now, there exists a variety of results which show that a wide range of functions can be approximated with sometimes surprising accuracy by these outputs. For example, it is known that the set of functions that can be approximated with exponential accuracy (in terms of the number of parameters used) includes, on one hand, very smooth functions such as polynomials and analytic functions (see e.g. \cite{E,S,Y}) and, on the other hand, very rough functions such as the Weierstrass function (see e.g. \cite{EPGB,DDFHP}), which is nowhere differentiable. In this paper, we add to the latter class of rough functions by showing that it also includes refinable functions. Namely, we show that refinable functions are approximated by the outputs of deep ReLU networks with a fixed width and increasing depth with accuracy exponential in terms of their number of parameters. Our results apply to functions used in the standard construction of wavelets as well as to functions constructed via subdivision algorithms in Computer Aided Geometric Design.
Type
Department
Description
Provenance
Subjects
Citation
Permalink
Collections
Scholars@Duke
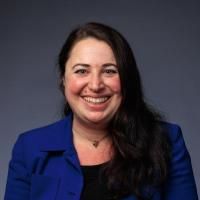
Shira Faigenbaum-Golovin
I am a Phillip Griffiths Assistant Research Professor at Duke University's math department as well as at the Rhodes Interdisciplinary Initiative, working with Prof. Ingrid Daubechies. In 2021 I completed my Ph.D. at the Department of Applied Mathematics, School of Mathematical Sciences, Tel Aviv University, under the supervision of Prof. David Levin and Prof. Yoel Shkolnisky.
My research interests span several areas, including numerical analysis, mathematical modeling, robust and statistically significant analysis of high-dimensional data. I strive to explore new challenges that arise from high-dimensional data as well as study the story that the data geometry tells by modeling the data and posing new mathematical tools. In particular, my research is in approximation theory in low and high-dimensions, geometric methods for manifold reconstruction, studying the geometry of the base manifold and its fibers, computer vision, image processing.
Notable applications of my current and past research include archaeology, evolutionary anthropology, Bible studies, art investigation, and general history. By applying my research to these diverse areas, I aim to contribute valuable insights and shed light on long debated questions.
My publication list (and most online available papers) can be viewed on Google Scholar.
Personal site: https://services.math.duke.edu/~ag617/
Unless otherwise indicated, scholarly articles published by Duke faculty members are made available here with a CC-BY-NC (Creative Commons Attribution Non-Commercial) license, as enabled by the Duke Open Access Policy. If you wish to use the materials in ways not already permitted under CC-BY-NC, please consult the copyright owner. Other materials are made available here through the author’s grant of a non-exclusive license to make their work openly accessible.