A miRNA Host Response Signature Accurately Discriminates Acute Respiratory Infection Etiologies.
Date
2018-01
Journal Title
Journal ISSN
Volume Title
Repository Usage Stats
views
downloads
Citation Stats
Abstract
Background: Acute respiratory infections (ARIs) are the leading indication for antibacterial prescriptions despite a viral etiology in the majority of cases. The lack of available diagnostics to discriminate viral and bacterial etiologies contributes to this discordance. Recent efforts have focused on the host response as a source for novel diagnostic targets although none have explored the ability of host-derived microRNAs (miRNA) to discriminate between these etiologies. Methods: In this study, we compared host-derived miRNAs and mRNAs from human H3N2 influenza challenge subjects to those from patients with Streptococcus pneumoniae pneumonia. Sparse logistic regression models were used to generate miRNA signatures diagnostic of ARI etiologies. Generalized linear modeling of mRNAs to identify differentially expressed (DE) genes allowed analysis of potential miRNA:mRNA relationships. High likelihood miRNA:mRNA interactions were examined using binding target prediction and negative correlation to further explore potential changes in pathway regulation in response to infection. Results: The resultant miRNA signatures were highly accurate in discriminating ARI etiologies. Mean accuracy was 100% [88.8-100; 95% Confidence Interval (CI)] in discriminating the healthy state from S. pneumoniae pneumonia and 91.3% (72.0-98.9; 95% CI) in discriminating S. pneumoniae pneumonia from influenza infection. Subsequent differential mRNA gene expression analysis revealed alterations in regulatory networks consistent with known biology including immune cell activation and host response to viral infection. Negative correlation network analysis of miRNA:mRNA interactions revealed connections to pathways with known immunobiology such as interferon regulation and MAP kinase signaling. Conclusion: We have developed novel human host-response miRNA signatures for bacterial and viral ARI etiologies. miRNA host response signatures reveal accurate discrimination between S. pneumoniae pneumonia and influenza etiologies for ARI and integrated analyses of the host-pathogen interface are consistent with expected biology. These results highlight the differential miRNA host response to bacterial and viral etiologies of ARI, offering new opportunities to distinguish these entities.
Type
Department
Description
Provenance
Citation
Permalink
Published Version (Please cite this version)
Publication Info
Poore, Gregory D, Emily R Ko, Ashlee Valente, Ricardo Henao, Kelsey Sumner, Christopher Hong, Thomas W Burke, Marshall Nichols, et al. (2018). A miRNA Host Response Signature Accurately Discriminates Acute Respiratory Infection Etiologies. Frontiers in microbiology, 9(DEC). p. 2957. 10.3389/fmicb.2018.02957 Retrieved from https://hdl.handle.net/10161/21657.
This is constructed from limited available data and may be imprecise. To cite this article, please review & use the official citation provided by the journal.
Collections
Scholars@Duke
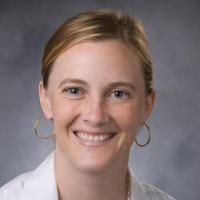
Emily Ray Ko
Clinical and translational research, COVID-19 therapeutics, clinical biomarkers for infectious disease.

Ricardo Henao
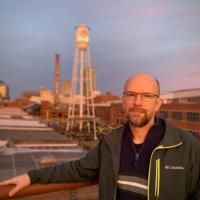
Thomas Burke
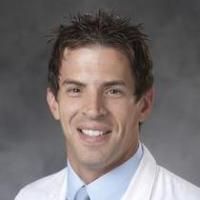
Micah Thomas McClain

Erich Senin Huang
Former Chief Data Officer for Quality, Duke Health
Former Director of Duke Forge
Former Director of Duke Crucible
Former Assistant Dean for Biomedical Informatics
Dr. Huang is currently Chief Science & Innovation Officer for Onduo by Verily, and Head of Clinical Informatics at Verily (Google's life sciences subsidiary), and is now adjunct faculty at Duke. Dr. Huang’s research interests span applied machine learning, research provenance and data infrastructure. Projects include building data provenance tools funded by the NIH’s Big Data to Knowledge program, regulatory science funded by the Burroughs Wellcome Foundation. Applied machine learning applications include “Deep Care Management” a highly interdisciplinary project with Duke Connected Care, Duke’s Accountable Care Organization, that integrates claims and EHR data for predicting unplanned admissions and risk stratifying patients for case management; CALYPSO, a collaboration with the Department of Surgery for utilizing machine learning to predict surgical complications. My team is also building the data platform for the Department of Surgery's "1000 Patients Project" an intensive biospecimen and biomarker study based around patients undergoing the controlled injury of surgery.
As Director of Duke Forge, Dr. Huang is working to build a data science culture and infrastructure across Duke University that focuses on actionable health data science. The Forge emphasizes scientific rigor, awareness that technology does not supersede clinicians’ responsibilities and human relationship with their patients, and the role of data science in society.

Geoffrey Steven Ginsburg
Dr. Geoffrey S. Ginsburg's research interests are in the development of novel paradigms for developing and translating genomic information into medical practice and the integration of personalized medicine into health care.

Christopher Wildrick Woods
1. Emerging Infections
2. Global Health
3. Epidemiology of infectious diseases
4. Clinical microbiology and diagnostics
5. Bioterrorism Preparedness
6. Surveillance for communicable diseases
7. Antimicrobial resistance
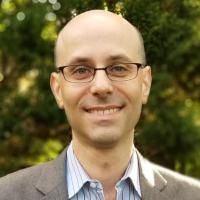
Ephraim Tsalik
My research at Duke has focused on understanding the dynamic between host and pathogen so as to discover and develop host-response markers that can diagnose and predict health and disease. This new and evolving approach to diagnosing illness has the potential to significantly impact individual as well as public health considering the rise of antibiotic resistance.
With any potential infectious disease diagnosis, it is difficult, if not impossible, to determine at the time of presentation what the underlying cause of illness is. For example, acute respiratory illness is among the most frequent reasons for patients to seek care. These symptoms, such as cough, sore throat, and fever may be due to a bacterial infection, viral infection, both, or a non-infectious condition such as asthma or allergies. Given the difficulties in making the diagnosis, most patients are inappropriately given antibacterials. However, each of these etiologies (bacteria, virus, or something else entirely) leaves a fingerprint embedded in the host’s response. We are very interested in finding those fingerprints and exploiting them to generate new approaches to understand, diagnose, and manage disease.
These principles also apply to sepsis, defined as life-threatening organ dysfunction caused by a dysregulated host response to infection. Just as with acute respiratory illness, it is often difficult to identify whether infection is responsible for a patient’s critical illness. We have embarked on a number of research programs that aim to better identify sepsis; define sepsis subtypes that can be used to guide future clinical research; and to better predict sepsis outcomes. These efforts have focused on many systems biology modalities including transcriptomics, miRNA, metabolomics, and proteomics. Consequently, our Data Science team has utilized these highly complex data to develop new statistical methods, furthering both the clinical and statistical research communities.
These examples are just a small sampling of the breadth of research Dr. Tsalik and his colleagues have conducted.
In April 2022, Dr. Tsalik has joined Danaher Diagnostics as the VP and Chief Scientific Officer for Infectious Disease, where he is applying this experience in biomarkers and diagnostics to shape the future of diagnostics in ID.
Unless otherwise indicated, scholarly articles published by Duke faculty members are made available here with a CC-BY-NC (Creative Commons Attribution Non-Commercial) license, as enabled by the Duke Open Access Policy. If you wish to use the materials in ways not already permitted under CC-BY-NC, please consult the copyright owner. Other materials are made available here through the author’s grant of a non-exclusive license to make their work openly accessible.