Reactive trajectories and the transition path process
Date
2014-01-01
Journal Title
Journal ISSN
Volume Title
Repository Usage Stats
views
downloads
Citation Stats
Abstract
© 2014, Springer-Verlag Berlin Heidelberg.We study the trajectories of a solution (formula presented) to an Itô stochastic differential equation in (formula presented), as the process passes between two disjoint open sets, (formula presented) and (formula presented). These segments of the trajectory are called transition paths or reactive trajectories, and they are of interest in the study of chemical reactions and thermally activated processes. In that context, the sets (formula presented) and (formula presented) represent reactant and product states. Our main results describe the probability law of these transition paths in terms of a transition path process (formula presented), which is a strong solution to an auxiliary SDE having a singular drift term. We also show that statistics of the transition path process may be recovered by empirical sampling of the original process (formula presented). As an application of these ideas, we prove various representation formulas for statistics of the transition paths. We also identify the density and current of transition paths. Our results fit into the framework of the transition path theory by Weinan and Vanden-Eijnden.
Type
Department
Description
Provenance
Subjects
Citation
Permalink
Published Version (Please cite this version)
Publication Info
Lu, J, and J Nolen (2014). Reactive trajectories and the transition path process. Probability Theory and Related Fields, 161(1-2). pp. 195–244. 10.1007/s00440-014-0547-y Retrieved from https://hdl.handle.net/10161/14092.
This is constructed from limited available data and may be imprecise. To cite this article, please review & use the official citation provided by the journal.
Collections
Scholars@Duke

Jianfeng Lu
Jianfeng Lu is an applied mathematician interested in mathematical analysis and algorithm development for problems from computational physics, theoretical chemistry, materials science, machine learning, and other related fields.
More specifically, his current research focuses include:
High dimensional PDEs; generative models and sampling methods; control and reinforcement learning; electronic structure and many body problems; quantum molecular dynamics; multiscale modeling and analysis.
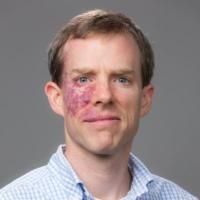
James H. Nolen
My research is in the area of probability and partial differential equations, which have been used to model many phenomena in the natural sciences and engineering. Asymptotic analysis has been a common theme in much of my research. Current research interests include: stochastic dynamics, interacting particle systems, reaction-diffusion equations, applications to biological models.
Unless otherwise indicated, scholarly articles published by Duke faculty members are made available here with a CC-BY-NC (Creative Commons Attribution Non-Commercial) license, as enabled by the Duke Open Access Policy. If you wish to use the materials in ways not already permitted under CC-BY-NC, please consult the copyright owner. Other materials are made available here through the author’s grant of a non-exclusive license to make their work openly accessible.