A Dynamic Directional Model for Effective Brain Connectivity using Electrocorticographic (ECoG) Time Series.
Date
2015-03-01
Journal Title
Journal ISSN
Volume Title
Repository Usage Stats
views
downloads
Citation Stats
Abstract
We introduce a dynamic directional model (DDM) for studying brain effective connectivity based on intracranial electrocorticographic (ECoG) time series. The DDM consists of two parts: a set of differential equations describing neuronal activity of brain components (state equations), and observation equations linking the underlying neuronal states to observed data. When applied to functional MRI or EEG data, DDMs usually have complex formulations and thus can accommodate only a few regions, due to limitations in spatial resolution and/or temporal resolution of these imaging modalities. In contrast, we formulate our model in the context of ECoG data. The combined high temporal and spatial resolution of ECoG data result in a much simpler DDM, allowing investigation of complex connections between many regions. To identify functionally segregated sub-networks, a form of biologically economical brain networks, we propose the Potts model for the DDM parameters. The neuronal states of brain components are represented by cubic spline bases and the parameters are estimated by minimizing a log-likelihood criterion that combines the state and observation equations. The Potts model is converted to the Potts penalty in the penalized regression approach to achieve sparsity in parameter estimation, for which a fast iterative algorithm is developed. The methods are applied to an auditory ECoG dataset.
Type
Department
Description
Provenance
Subjects
Citation
Permalink
Published Version (Please cite this version)
Publication Info
Zhang, Tingting, Jingwei Wu, Fan Li, Brian Caffo and Dana Boatman-Reich (2015). A Dynamic Directional Model for Effective Brain Connectivity using Electrocorticographic (ECoG) Time Series. J Am Stat Assoc, 110(509). pp. 93–106. 10.1080/01621459.2014.988213 Retrieved from https://hdl.handle.net/10161/10304.
This is constructed from limited available data and may be imprecise. To cite this article, please review & use the official citation provided by the journal.
Collections
Scholars@Duke
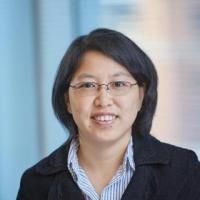
Fan Li
My main research interest is causal inference and its applications to health, policy and social science. I also work on the interface between causal inference and machine learning. I have developed methods for propensity score, clinical trials, randomized experiments (e.g. A/B testing), difference-in-differences, regression discontinuity designs, representation learning. I also work on Bayesian analysis and statistical methods for missing data. I am serving as the editor for social science, biostatistics and policy for the journal Annals of Applied Statistics.
Unless otherwise indicated, scholarly articles published by Duke faculty members are made available here with a CC-BY-NC (Creative Commons Attribution Non-Commercial) license, as enabled by the Duke Open Access Policy. If you wish to use the materials in ways not already permitted under CC-BY-NC, please consult the copyright owner. Other materials are made available here through the author’s grant of a non-exclusive license to make their work openly accessible.