An Ensemble Approach to Knowledge-Based Intensity-Modulated Radiation Therapy Planning.
Date
2018-01
Journal Title
Journal ISSN
Volume Title
Repository Usage Stats
views
downloads
Citation Stats
Attention Stats
Abstract
Knowledge-based planning (KBP) utilizes experienced planners' knowledge embedded in prior plans to estimate optimal achievable dose volume histogram (DVH) of new cases. In the regression-based KBP framework, previously planned patients' anatomical features and DVHs are extracted, and prior knowledge is summarized as the regression coefficients that transform features to organ-at-risk DVH predictions. In our study, we find that in different settings, different regression methods work better. To improve the robustness of KBP models, we propose an ensemble method that combines the strengths of various linear regression models, including stepwise, lasso, elastic net, and ridge regression. In the ensemble approach, we first obtain individual model prediction metadata using in-training-set leave-one-out cross validation. A constrained optimization is subsequently performed to decide individual model weights. The metadata is also used to filter out impactful training set outliers. We evaluate our method on a fresh set of retrospectively retrieved anonymized prostate intensity-modulated radiation therapy (IMRT) cases and head and neck IMRT cases. The proposed approach is more robust against small training set size, wrongly labeled cases, and dosimetric inferior plans, compared with other individual models. In summary, we believe the improved robustness makes the proposed method more suitable for clinical settings than individual models.
Type
Department
Description
Provenance
Subjects
Citation
Permalink
Published Version (Please cite this version)
Publication Info
Zhang, Jiahan, Q Jackie Wu, Tianyi Xie, Yang Sheng, Fang-Fang Yin and Yaorong Ge (2018). An Ensemble Approach to Knowledge-Based Intensity-Modulated Radiation Therapy Planning. Frontiers in oncology, 8(MAR). p. 57. 10.3389/fonc.2018.00057 Retrieved from https://hdl.handle.net/10161/19374.
This is constructed from limited available data and may be imprecise. To cite this article, please review & use the official citation provided by the journal.
Collections
Scholars@Duke

Qingrong Jackie Wu
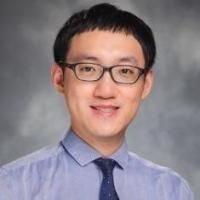
Yang Sheng
My research interest focuses on machine learning and AI application in radiation oncology treatment planning, including prostate cancer, head-and-neck cancer and pancreatic cancer etc.
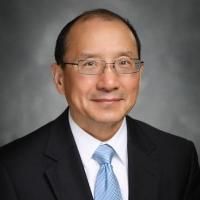
Fang-Fang Yin
Stereotactic radiosurgery, Stereotactic body radiation therapy, treatment planning optimization, knowledge guided radiation therapy, intensity-modulated radiation therapy, image-guided radiation therapy, oncological imaging and informatics
Unless otherwise indicated, scholarly articles published by Duke faculty members are made available here with a CC-BY-NC (Creative Commons Attribution Non-Commercial) license, as enabled by the Duke Open Access Policy. If you wish to use the materials in ways not already permitted under CC-BY-NC, please consult the copyright owner. Other materials are made available here through the author’s grant of a non-exclusive license to make their work openly accessible.