Component-wise gradient boosting and false discovery control in survival analysis with high-dimensional covariates.
Date
2016-01-01
Journal Title
Journal ISSN
Volume Title
Repository Usage Stats
views
downloads
Citation Stats
Attention Stats
Abstract
MOTIVATION: Technological advances that allow routine identification of high-dimensional risk factors have led to high demand for statistical techniques that enable full utilization of these rich sources of information for genetics studies. Variable selection for censored outcome data as well as control of false discoveries (i.e. inclusion of irrelevant variables) in the presence of high-dimensional predictors present serious challenges. This article develops a computationally feasible method based on boosting and stability selection. Specifically, we modified the component-wise gradient boosting to improve the computational feasibility and introduced random permutation in stability selection for controlling false discoveries. RESULTS: We have proposed a high-dimensional variable selection method by incorporating stability selection to control false discovery. Comparisons between the proposed method and the commonly used univariate and Lasso approaches for variable selection reveal that the proposed method yields fewer false discoveries. The proposed method is applied to study the associations of 2339 common single-nucleotide polymorphisms (SNPs) with overall survival among cutaneous melanoma (CM) patients. The results have confirmed that BRCA2 pathway SNPs are likely to be associated with overall survival, as reported by previous literature. Moreover, we have identified several new Fanconi anemia (FA) pathway SNPs that are likely to modulate survival of CM patients. AVAILABILITY AND IMPLEMENTATION: The related source code and documents are freely available at https://sites.google.com/site/bestumich/issues. CONTACT: yili@umich.edu.
Type
Department
Description
Provenance
Subjects
Citation
Permalink
Published Version (Please cite this version)
Publication Info
He, Kevin, Yanming Li, Ji Zhu, Hongliang Liu, Jeffrey E Lee, Christopher I Amos, Terry Hyslop, Jiashun Jin, et al. (2016). Component-wise gradient boosting and false discovery control in survival analysis with high-dimensional covariates. Bioinformatics, 32(1). pp. 50–57. 10.1093/bioinformatics/btv517 Retrieved from https://hdl.handle.net/10161/10678.
This is constructed from limited available data and may be imprecise. To cite this article, please review & use the official citation provided by the journal.
Collections
Scholars@Duke
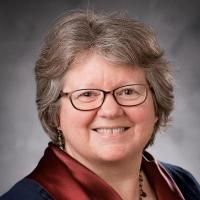
Terry Hyslop

Qingyi Wei
Qingyi Wei, MD, PhD, Professor in the Department of Medicine, is Associate Director for Cancer Control and Population Sciences, Co-leader of CCPS and Co-leader of Epidemiology and Population Genomics (Focus Area 1). He is a professor of Medicine and an internationally recognized epidemiologist focused on the molecular and genetic epidemiology of head and neck cancers, lung cancer, and melanoma. His research focuses on biomarkers and genetic determinants for the DNA repair deficient phenotype and variations in cell death. He is Editor-in-Chief of the open access journal "Cancer Medicine" and Associate Editor-in-Chief of the International Journal of Molecular Epidemiology and Genetics.
Area of Expertise: Epidemiology
Unless otherwise indicated, scholarly articles published by Duke faculty members are made available here with a CC-BY-NC (Creative Commons Attribution Non-Commercial) license, as enabled by the Duke Open Access Policy. If you wish to use the materials in ways not already permitted under CC-BY-NC, please consult the copyright owner. Other materials are made available here through the author’s grant of a non-exclusive license to make their work openly accessible.