A branching process model for flow cytometry and budding index measurements in cell synchrony experiments.
Date
2009
Journal Title
Journal ISSN
Volume Title
Repository Usage Stats
views
downloads
Citation Stats
Abstract
We present a flexible branching process model for cell population dynamics in synchrony/time-series experiments used to study important cellular processes. Its formulation is constructive, based on an accounting of the unique cohorts in the population as they arise and evolve over time, allowing it to be written in closed form. The model can attribute effects to subsets of the population, providing flexibility not available using the models historically applied to these populations. It provides a tool for in silico synchronization of the population and can be used to deconvolve population-level experimental measurements, such as temporal expression profiles. It also allows for the direct comparison of assay measurements made from multiple experiments. The model can be fit either to budding index or DNA content measurements, or both, and is easily adaptable to new forms of data. The ability to use DNA content data makes the model applicable to almost any organism. We describe the model and illustrate its utility and flexibility in a study of cell cycle progression in the yeast Saccharomyces cerevisiae.
Type
Department
Description
Provenance
Subjects
Citation
Permalink
Published Version (Please cite this version)
Publication Info
Orlando, David A, Edwin S Iversen, Alexander J Hartemink and Steven B Haase (2009). A branching process model for flow cytometry and budding index measurements in cell synchrony experiments. Ann Appl Stat, 3(4). pp. 1521–1541. 10.1214/09-AOAS264 Retrieved from https://hdl.handle.net/10161/13267.
This is constructed from limited available data and may be imprecise. To cite this article, please review & use the official citation provided by the journal.
Collections
Scholars@Duke

Edwin Severin Iversen
Bayesian statistical modeling with application to problems in genetic
epidemiology and cancer research; models for epidemiological risk
assessment, including hierarchical methods for combining related
epidemiological studies; ascertainment corrections for high risk
family data; analysis of high-throughput genomic data sets.
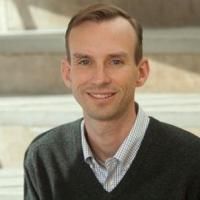
Alexander J. Hartemink
Computational biology, machine learning, Bayesian statistics, transcriptional regulation, genomics and epigenomics, graphical models, Bayesian networks, hidden Markov models, systems biology, computational neurobiology, classification, feature selection
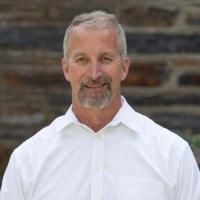
Steven B. Haase
Our group is broadly interested in understanding the biological clock mechanisms that control the timing of events during the cell division cycle. In 2008, the Haase group proposed a new model in which a complex network of sequentially activated transcription factors regulates the precise timing of gene expression during the cell-cycle, and functions as a robust time-keeping oscillator. Greater than a thousand genes are expressed at distinct phases of the cycle, and the control network itself consists of ~20 components, so this dynamical system is far too complex to understand simply by biological intuition. We rely heavily on the expertise of the Harer group (Dept. of Mathematics, Duke University) for the analysis of complex data, and their understanding of dynamical systems. Using a collection of tools, including molecular genetics, genomics, mathematical models, and statistical inference, our groups aim to understand how the cell division clock works, how it might be perturbed in proliferative diseases such as cancer, and how the clock components might be targeted for new anti-tumor therapies. Qualitatively, the clock networks that control the yeast cell cycle look much like the networks controlling circadian rhythms in a variety of organisms. More recently, we have been using our experimental and quantitative approaches to investigate the function of circadian clocks, as well as clocks that control the division and development of pathogenic organisms such as P. falciparum and P. vivax, the causative agents of malaria.
Unless otherwise indicated, scholarly articles published by Duke faculty members are made available here with a CC-BY-NC (Creative Commons Attribution Non-Commercial) license, as enabled by the Duke Open Access Policy. If you wish to use the materials in ways not already permitted under CC-BY-NC, please consult the copyright owner. Other materials are made available here through the author’s grant of a non-exclusive license to make their work openly accessible.