Whole-slide cytometric feature mapping for distinguishing tumor genomic subtypes in HNSCC whole slide images.
Date
2022-11
Journal Title
Journal ISSN
Volume Title
Repository Usage Stats
views
downloads
Citation Stats
Attention Stats
Abstract
Head and neck squamous cell carcinoma (HNSCC) is a heterogenous disease where, in advanced stages, clinical and pathological stages do not correlate with outcome. Molecular and genomic biomarkers for HNSCC classification have shown promise for prognostic and therapeutic applications. In this study, we utilize automated image analysis techniques in whole slide images of HNSCC tumors to identify relationships between cytometric features and genomic phenotypes. Hematoxylin and eosin-stained slides of HNSCC tumors (N=49) were obtained from the Cancer Imaging Archive (TCIA), along with accompanying clinical, pathological, genomic, and proteomic reports. Automated nuclear detection was performed across the entirety of slides, and cytometric feature maps were generated. Forty-one cytometric features were evaluated for associations with tumor grade, tumor stage, tumor subsite, and integrated genomic subtype (IGS). Thirty-two features demonstrated significant association with IGS when corrected for multiple comparisons. In particular, the basal subtype was visually distinguishable from the chromosomal instability and immune subtypes based on cytometric feature measurements. No features were significantly associated with tumor grade, stage, or subsite. This study provides preliminary evidence that features derived from tissue pathology slides could provide insights into genomic phenotypes of HNSCC.
Type
Department
Description
Provenance
Subjects
Citation
Permalink
Published Version (Please cite this version)
Publication Info
Blocker, Stephanie J, Samantha Morrison, Jeffrey I Everitt, James Cook, Sheng Luo, Tammara L Watts and Yvonne M Mowery (2022). Whole-slide cytometric feature mapping for distinguishing tumor genomic subtypes in HNSCC whole slide images. The American journal of pathology. p. S0002-9440(22)00362-5. 10.1016/j.ajpath.2022.11.004 Retrieved from https://hdl.handle.net/10161/26262.
This is constructed from limited available data and may be imprecise. To cite this article, please review & use the official citation provided by the journal.
Collections
Scholars@Duke
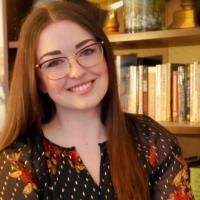
Stephanie Blocker
I am a cancer biologist whose research laboratory focuses on solid tumor imaging. We utilize multi-modal, multi-scale imaging, combined with nuanced statistical and machine learning approaches, to measure important features of cancer. My goal os to develop and translate imaging approaches which improve clinical diagnostics and personalize care for cancer patients.

Samantha Morrison
Education: PhD, Biostatistics -- Brown University, 2021
Samantha collaborates with clinicians and researchers in the Department of Radiology, the Duke Transplant Center, and the Division of Pulmonary, Allergy, and Critical Care Medicine in the Department of Medicine. Her research focuses on development and evaluation of prediction models in the presence of incomplete outcome data. Her areas of interest include machine learning methods and survival analysis.
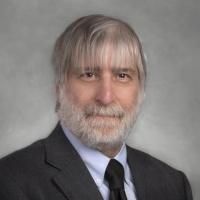
Jeffrey Ira Everitt
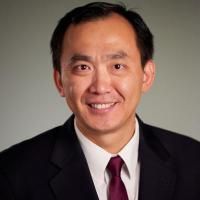
Sheng Luo

Tammara Lynn Watts
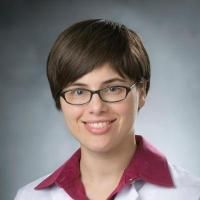
Yvonne Marie Mowery
Unless otherwise indicated, scholarly articles published by Duke faculty members are made available here with a CC-BY-NC (Creative Commons Attribution Non-Commercial) license, as enabled by the Duke Open Access Policy. If you wish to use the materials in ways not already permitted under CC-BY-NC, please consult the copyright owner. Other materials are made available here through the author’s grant of a non-exclusive license to make their work openly accessible.