Attack-Resilient State Estimation in the Presence of Noise
Abstract
We consider the problem of attack-resilient state estimation in the presence of noise. We focus on the most general model for sensor attacks where {any} signal can be injected via the compromised sensors. An $l_0$-based state estimator that can be formulated as a mixed-integer linear program and its convex relaxation based on the $l_1$ norm are presented. For both $l_0$ and $l_1$-based state estimators, we derive rigorous analytic bounds on the state-estimation errors. We show that the worst-case error is linear with the size of the noise, meaning that the attacker cannot exploit noise and modeling errors to introduce unbounded state-estimation errors. Finally, we show how the presented attack-resilient state estimators can be used for sound attack detection and identification, and provide conditions on the size of attack vectors that will ensure correct identification of compromised sensors.
Type
Department
Description
Provenance
Subjects
Citation
Permalink
Collections
Scholars@Duke
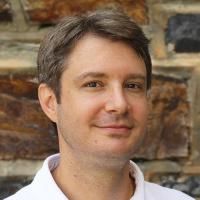
Miroslav Pajic
Miroslav Pajic's research focuses on design and analysis of cyber-physical systems with varying levels of autonomy and human interaction, at the intersection of (more traditional) areas of embedded systems, AI, learning and controls, formal methods and robotics.
Unless otherwise indicated, scholarly articles published by Duke faculty members are made available here with a CC-BY-NC (Creative Commons Attribution Non-Commercial) license, as enabled by the Duke Open Access Policy. If you wish to use the materials in ways not already permitted under CC-BY-NC, please consult the copyright owner. Other materials are made available here through the author’s grant of a non-exclusive license to make their work openly accessible.