Advances to Bayesian network inference for generating causal networks from observational biological data.
Date
2004-12-12
Journal Title
Journal ISSN
Volume Title
Repository Usage Stats
views
downloads
Citation Stats
Attention Stats
Abstract
MOTIVATION: Network inference algorithms are powerful computational tools for identifying putative causal interactions among variables from observational data. Bayesian network inference algorithms hold particular promise in that they can capture linear, non-linear, combinatorial, stochastic and other types of relationships among variables across multiple levels of biological organization. However, challenges remain when applying these algorithms to limited quantities of experimental data collected from biological systems. Here, we use a simulation approach to make advances in our dynamic Bayesian network (DBN) inference algorithm, especially in the context of limited quantities of biological data. RESULTS: We test a range of scoring metrics and search heuristics to find an effective algorithm configuration for evaluating our methodological advances. We also identify sampling intervals and levels of data discretization that allow the best recovery of the simulated networks. We develop a novel influence score for DBNs that attempts to estimate both the sign (activation or repression) and relative magnitude of interactions among variables. When faced with limited quantities of observational data, combining our influence score with moderate data interpolation reduces a significant portion of false positive interactions in the recovered networks. Together, our advances allow DBN inference algorithms to be more effective in recovering biological networks from experimentally collected data. AVAILABILITY: Source code and simulated data are available upon request. SUPPLEMENTARY INFORMATION: http://www.jarvislab.net/Bioinformatics/BNAdvances/
Type
Department
Description
Provenance
Subjects
Citation
Permalink
Published Version (Please cite this version)
Publication Info
Yu, Jing, V Anne Smith, Paul P Wang, Alexander J Hartemink and Erich D Jarvis (2004). Advances to Bayesian network inference for generating causal networks from observational biological data. Bioinformatics, 20(18). pp. 3594–3603. 10.1093/bioinformatics/bth448 Retrieved from https://hdl.handle.net/10161/11228.
This is constructed from limited available data and may be imprecise. To cite this article, please review & use the official citation provided by the journal.
Collections
Scholars@Duke
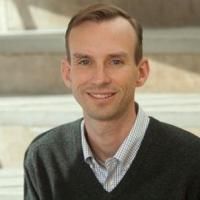
Alexander J. Hartemink
Computational biology, machine learning, Bayesian statistics, transcriptional regulation, genomics and epigenomics, graphical models, Bayesian networks, hidden Markov models, systems biology, computational neurobiology, classification, feature selection

Erich David Jarvis
Dr. Jarvis' laboratory studies the neurobiology of vocal communication. Emphasis is placed on the molecular pathways involved in the perception and production of learned vocalizations. They use an integrative approach that combines behavioral, anatomical, electrophysiological and molecular biological techniques. The main animal model used is songbirds, one of the few vertebrate groups that evolved the ability to learn vocalizations. The generality of the discoveries is tested in other vocal learning orders, such as parrots and hummingbirds, as well as non-vocal learners, such as pigeons and non-human primates. Some of the questions require performing behavior/molecular biology experiments in freely ranging animals, such as hummingbirds in tropical forest of Brazil. Recent results show that in songbirds, parrots and hummingbirds, perception and production of song are accompanied by anatomically distinct patterns of gene expression. All three groups were found to exhibit vocally-activated gene expression in exactly 7 forebrain nuclei that are very similar to each other. These structures for vocal learning and production are thought to have evolved independently within the past 70 million years, since they are absent from interrelated non-vocal learning orders. One structure, Area X of the basal ganglia's striatum in songbirds, shows large differential gene activation depending on the social context in which the bird sings. These differences may reflect a semantic content of song, perhaps similar to human language.
The overall goal of the research is to advance knowledge of the neural mechanisms for vocal learning and basic mechanisms of brain function. These goals are further achieved by combined collaborative efforts with the laboratories of Drs. Mooney and Nowicki at Duke University, who study respectively behavior and electrophysiological aspects of songbird vocal communication.
Unless otherwise indicated, scholarly articles published by Duke faculty members are made available here with a CC-BY-NC (Creative Commons Attribution Non-Commercial) license, as enabled by the Duke Open Access Policy. If you wish to use the materials in ways not already permitted under CC-BY-NC, please consult the copyright owner. Other materials are made available here through the author’s grant of a non-exclusive license to make their work openly accessible.