Application of Machine Learning in Pulmonary Function Assessment Where Are We Now and Where Are We Going?
Date
2021-01
Journal Title
Journal ISSN
Volume Title
Repository Usage Stats
views
downloads
Citation Stats
Attention Stats
Abstract
Analysis of pulmonary function tests (PFTs) is an area where machine learning (ML) may benefit clinicians, researchers, and the patients. PFT measures spirometry, lung volumes, and carbon monoxide diffusion capacity of the lung (DLCO). The results are usually interpreted by the clinicians using discrete numeric data according to published guidelines. PFT interpretations by clinicians, however, are known to have inter-rater variability and the inaccuracy can impact patient care. This variability may be caused by unfamiliarity of the guidelines, lack of training, inadequate understanding of lung physiology, or simply mental lapses. A rules-based automated interpretation system can recapitulate expert's pattern recognition capability and decrease errors. ML can also be used to analyze continuous data or the graphics, including the flow-volume loop, the DLCO and the nitrogen washout curves. These analyses can discover novel physiological biomarkers. In the era of wearables and telehealth, particularly with the COVID-19 pandemic restricting PFTs to be done in the clinical laboratories, ML can also be used to combine mobile spirometry results with an individual's clinical profile to deliver precision medicine. There are, however, hurdles in the development and commercialization of the ML-assisted PFT interpretation programs, including the need for high quality representative data, the existence of different formats for data acquisition and sharing in PFT software by different vendors, and the need for collaboration amongst clinicians, biomedical engineers, and information technologists. Hurdles notwithstanding, the new developments would represent significant advances that could be the future of PFT, the oldest test still in use in clinical medicine.
Type
Department
Description
Provenance
Citation
Permalink
Published Version (Please cite this version)
Publication Info
Giri, Paresh C, Anand M Chowdhury, Armando Bedoya, Hengji Chen, Hyun Suk Lee, Patty Lee, Craig Henriquez, Neil R MacIntyre, et al. (2021). Application of Machine Learning in Pulmonary Function Assessment Where Are We Now and Where Are We Going?. Frontiers in physiology, 12. p. 678540. 10.3389/fphys.2021.678540 Retrieved from https://hdl.handle.net/10161/25609.
This is constructed from limited available data and may be imprecise. To cite this article, please review & use the official citation provided by the journal.
Collections
Scholars@Duke
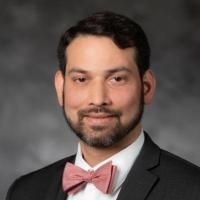
Ananda Chowdhury

Armando Diego Bedoya

Neil Ross MacIntyre
- Mechanical Ventilation and respiratory failure. Current projects involve studying patient-ventilator interactions during modes of support that require patient activity. The focus is on ventilatory muscle function during these assisted modes. Other projects include evaluating respiratory system mechanics in acute respiratory failure and the role of ECMO in the adult. Duke is also one of several institutions in the NIH ARDS Network, a consortium designed to perform multi-center trials.
2) Diffusing capacity of the lung for carbon monoxide. Current projects involve using a rapidly responding gas analyzer to measure lung diffusing capacity in discrete regions of the lung. These analyses are being extended into mechanically ventilated patients.
3) COPD. Current projects involve studying the physiology of cardio-respiratory conditioning in patients with obstructive lung disease and the role of pulmonary rehabilitation in improving COPD outcomes. Duke is also a member of two NIH sponsored multicenter programs: the COPDgene project correlating genetic profiles with physiologic/clinical phenotypes; and the NIH LOTT project evaluating the role of oxygen therapy in exercise and sleep hypoxemia.
4) Aerosol delivery systems. The current project is the development of a prototype aerosol generating catheter that can be directly inserted into the airways. Studies are being conducted in both ex vivo animal lungs and in mechanically ventilated patients.

Yuh-Chin Tony Huang
Closed loop ventilation
Environmental medicine
Oxidative lung injury
COPD
Hyperpolarized 129Xe MRI and regional lung function
Unless otherwise indicated, scholarly articles published by Duke faculty members are made available here with a CC-BY-NC (Creative Commons Attribution Non-Commercial) license, as enabled by the Duke Open Access Policy. If you wish to use the materials in ways not already permitted under CC-BY-NC, please consult the copyright owner. Other materials are made available here through the author’s grant of a non-exclusive license to make their work openly accessible.