Automated quality control in nuclear medicine using the structured noise index.
Date
2020-04
Authors
Journal Title
Journal ISSN
Volume Title
Repository Usage Stats
views
downloads
Citation Stats
Attention Stats
Abstract
Purpose
Daily flood-field uniformity evaluation serves as the central element of nuclear medicine (NM) quality control (QC) programs. Uniformity images are traditionally analyzed using pixel value-based metrics, that is, integral uniformity (IU), which often fail to capture subtle structure and patterns caused by changes in gamma camera performance, requiring visual inspections which are subjective and time demanding. The goal of this project was to implement an advanced QC metrology for NM to effectively identify nonuniformity issues, and report issues in a timely manner for efficient correction prior to clinical use. The project involved the implementation of the program over a 2-year period at a multisite major medical institution.Methods
Using a previously developed quantitative uniformity analysis metric, the structured noise index (SNI) [Nelson et al. (2014), \textit{J Nucl Med.}, \textbf{55}:169-174], an automated QC process was developed to analyze, archive, and report on daily NM QC uniformity images. Clinical implementation of the newly developed program ran in parallel with the manufacturer's reported IU-based QC program. The effectiveness of the SNI program was evaluated over a 21-month period using sensitivity and coefficient of variation statistics.Results
A total of 7365 uniformity QC images were analyzed. Lower level SNI alerts were generated in 12.5% of images and upper level alerts in 1.7%. Intervention due to image quality issues occurred on 26 instances; the SNI metric identified 24, while the IU metric identified eight. The SNI metric reported five upper level alerts where no clinical engineering intervention was deemed necessary.Conclusion
An SNI-based QC program provides a robust quantification of the performance of gamma camera uniformity. It operates seamlessly across a fleet of multiple camera models and, additionally, provides effective workflow among the clinical staff. The reliability of this process could eliminate the need for visual inspection of each image, saving valuable time, while enabling quantitative analysis of inter- and intrasystem performance.Type
Department
Description
Provenance
Subjects
Citation
Permalink
Published Version (Please cite this version)
Publication Info
Nelson, Jeffrey S, and Ehsan Samei (2020). Automated quality control in nuclear medicine using the structured noise index. Journal of applied clinical medical physics, 21(4). pp. 80–86. 10.1002/acm2.12850 Retrieved from https://hdl.handle.net/10161/26095.
This is constructed from limited available data and may be imprecise. To cite this article, please review & use the official citation provided by the journal.
Collections
Scholars@Duke
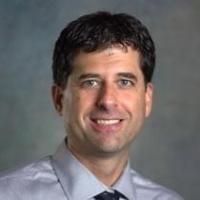
Jeffrey Nelson

Ehsan Samei
Dr. Ehsan Samei, PhD, DABR, FAAPM, FSPIE, FAIMBE, FIOMP, FACR is a Persian-American medical physicist. He is the Reed and Martha Rice Distinguished Professor of Radiology, and Professor of Medical Physics, Biomedical Engineering, Physics, and Electrical and Computer Engineering at Duke University. He serves as the Chief Imaging Physicist for Duke University Health System, the Director of the Carl E Ravin Advanced Imaging Laboratories and the Center for Virtual Imaging Trials (CVIT), and co-PI of one the five Centers of Excellence in Regulatory Science and Innovation (CERSI), Triangle CERSI. He is certified by the American Board of Radiology, recognized as a Distinguished Investigator by the Academy of Radiology Research, and awarded Fellow by five professional organization. He founded/co-founded the Duke Medical Physics Program, the Duke Imaging Physics Residency Program, the Duke Clinical Imaging Physics Group, the Center for Virtual Imaging Trials, and the Society of Directors of Academic Medical Physics Programs (SDAMPP). He has held senior leadership positions in the AAPM, SPIE, SDAMPP, and RSNA, including election to the presidency of the SEAAPM (2010-2011), SDAMPP (2011), and AAPM (2023).
Dr. Samei's scientific expertise include x-ray imaging, theoretical imaging models, simulation methods, and experimental techniques in medical image formation, quantification, and perception. His research aims to bridge the gap between scientific scholarship and clinical practice, in the meaningful realization of translational research, and in clinical processes that are informed by scientific evidence. He has advanced image quality and safety metrics and radiometrics that are clinically relevant and that can be used to design, optimize, and monitor interpretive and quantitative performance of imaging techniques. These have been implemented in advanced imaging performance characterization, procedural optimization, and clinical dose and quality analytics. His most recent research interests have been virtual clinical trial across a broad spectrum of oncologic, pulmonary, cardiac, and vascular diseases, and developing methodological advances that provide smart fusions of principle-informed and AI-based, data-informed approaches to scientific inquiry.
Dr. Samei has mentored over 140 trainees (graduate and postgraduate). He has more than 1400 scientific publications including more than 360 referred journal articles, 600 conference presentations, and 4 books. Citations to his work is reflected in an h-index of 74 and a Weighted Relative Citation Ratio of 613. His laboratory of over 20 researchers has been supported continuously over two decades by 44 extramural grants, culminating in a NIH Program Project grant in 2021 to establish the national Center for Virtual Imaging Trials (CVIT), joining a small number of prominent Biomedical Technology Research Centers across the nation. In 2023, he, along with 3 other PIs, was awarded to lead one of five national Centers of Excellence in Regulatory Science and Innovation (Triangle CERSI) by the FDA.
Unless otherwise indicated, scholarly articles published by Duke faculty members are made available here with a CC-BY-NC (Creative Commons Attribution Non-Commercial) license, as enabled by the Duke Open Access Policy. If you wish to use the materials in ways not already permitted under CC-BY-NC, please consult the copyright owner. Other materials are made available here through the author’s grant of a non-exclusive license to make their work openly accessible.