Evaluating renal lesions using deep-learning based extension of dual-energy FoV in dual-source CT-A retrospective pilot study.
Date
2021-06
Journal Title
Journal ISSN
Volume Title
Repository Usage Stats
views
downloads
Citation Stats
Attention Stats
Abstract
Purpose
Dual-source (DS) CT, dual-energy (DE) field of view (FoV) is limited to the size of the smaller detector array. The purpose was to establish a deep learning-based approach to DE extrapolation by estimating missing image data using data from both tubes to evaluate renal lesions.Method
A DE extrapolation deep-learning (DEEDL) algorithm had been trained on DECT data of 50 patients using a DSCT with DE-FoV = 33 cm (Somatom Flash). Data from 128 patients with known renal lesions falling within DE-FoV was retrospectively collected (100/140 kVp; reference dataset 1). A smaller DE-FoV = 20 cm was simulated excluding the renal lesion of interest (dataset 2) and the DEEDL was applied to this dataset. Output from the DEEDL algorithm was evaluated using ReconCT v14.1 and Syngo.via. Mean attenuation values in lesions on mixed images (HU) were compared calculating the root-mean-squared-error (RMSE) between the datasets using MATLAB R2019a.Results
The DEEDL algorithm performed well reproducing the image data of the kidney lesions (Bosniak 1 and 2: 125, Bosniak 2F: 6, Bosniak 3: 1 and Bosniak 4/(partially) solid: 32) with RSME values of 10.59 HU, 15.7 HU for attenuation, virtual non-contrast, respectively. The measurements performed in dataset 1 and 2 showed strong correlation with linear regression (r2: attenuation = 0.89, VNC = 0.63, iodine = 0.75), lesions were classified as enhancing with an accuracy of 0.91.Conclusion
This DEEDL algorithm can be used to reconstruct a full dual-energy FoV from restricted data, enabling reliable HU value measurements in areas not covered by the smaller FoV and evaluation of renal lesions.Type
Department
Description
Provenance
Subjects
Citation
Permalink
Published Version (Please cite this version)
Publication Info
Schwartz, Fides R, Darin P Clark, Yuqin Ding, Juan Carlos Ramirez-Giraldo, Cristian T Badea and Daniele Marin (2021). Evaluating renal lesions using deep-learning based extension of dual-energy FoV in dual-source CT-A retrospective pilot study. European journal of radiology, 139. p. 109734. 10.1016/j.ejrad.2021.109734 Retrieved from https://hdl.handle.net/10161/24252.
This is constructed from limited available data and may be imprecise. To cite this article, please review & use the official citation provided by the journal.
Collections
Scholars@Duke
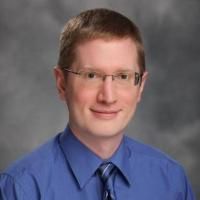
Darin Clark
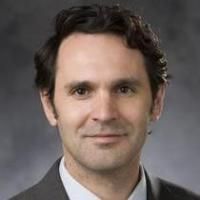
Cristian Tudorel Badea
- Our lab's research focus lies primarily in developing novel quantitative imaging systems, reconstruction algorithms and analysis methods. My major expertise is in preclinical CT.
- Currently, we are particularly interested in developing novel strategies for spectral CT imaging using nanoparticle-based contrast agents for theranostics (i.e. therapy and diagnostics).
- We are also engaged in developing new approaches for multidimensional CT image reconstruction suitable to address difficult undersampling cases in cardiac and spectral CT (dual energy and photon counting) using compressed sensing and/or deep learning.
- We are involved in co-clinical cancer trials and I have served as the Principal Investigator on the U24 Duke Preclinical Research Resources for Quantitative Imaging Biomarkers part of the NCI Co-Clinical Imaging Research Resources Program network (CIRP).
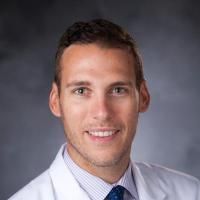
Daniele Marin
Liver Imaging
Dual Energy CT
CT Protocol Optimization
Dose Reduction Strategies for Abdominal CT Applications
Unless otherwise indicated, scholarly articles published by Duke faculty members are made available here with a CC-BY-NC (Creative Commons Attribution Non-Commercial) license, as enabled by the Duke Open Access Policy. If you wish to use the materials in ways not already permitted under CC-BY-NC, please consult the copyright owner. Other materials are made available here through the author’s grant of a non-exclusive license to make their work openly accessible.