Incorporating Case-Based Reasoning for Radiation Therapy Knowledge Modeling: A Pelvic Case Study.
Date
2019-01
Journal Title
Journal ISSN
Volume Title
Repository Usage Stats
views
downloads
Citation Stats
Abstract
Knowledge models in radiotherapy capture the relation between patient anatomy and dosimetry to provide treatment planning guidance. When treatment schemes evolve, existing models struggle to predict accurately. We propose a case-based reasoning framework designed to handle novel anatomies that are of same type but vary beyond original training samples. A total of 105 pelvic intensity-modulated radiotherapy cases were analyzed. Eighty cases were prostate cases while the other 25 were prostate-plus-lymph-node cases. We simulated 4 scenarios: Scarce scenario, Semiscarce scenario, Semiample scenario, and Ample scenario. For the Scarce scenario, a multiple stepwise regression model was trained using 85 cases (80 prostate, 5 prostate-plus-lymph-node). The proposed workflow started with evaluating the feature novelty of new cases against 5 training prostate-plus-lymph-node cases using leverage statistic. The case database was composed of a 5-case dose atlas. Case-based dose prediction was compared against the regression model prediction using sum of squared residual. Mean sum of squared residual of case-based and regression predictions for the bladder of 13 identified outliers were 0.174 ± 0.166 and 0.459 ± 0.508, respectively (P = .0326). For the rectum, the respective mean sum of squared residuals were 0.103 ± 0.120 and 0.150 ± 0.171 for case-based and regression prediction (P = .1972). By retaining novel cases, under the Ample scenario, significant statistical improvement was observed over the Scarce scenario (P = .0398) for the bladder model. We expect that the incorporation of case-based reasoning that judiciously applies appropriate predictive models could improve overall prediction accuracy and robustness in clinical practice.
Type
Department
Description
Provenance
Citation
Permalink
Published Version (Please cite this version)
Publication Info
Sheng, Yang, Jiahan Zhang, Chunhao Wang, Fang-Fang Yin, Q Jackie Wu and Yaorong Ge (2019). Incorporating Case-Based Reasoning for Radiation Therapy Knowledge Modeling: A Pelvic Case Study. Technology in cancer research & treatment, 18. p. 1533033819874788. 10.1177/1533033819874788 Retrieved from https://hdl.handle.net/10161/19363.
This is constructed from limited available data and may be imprecise. To cite this article, please review & use the official citation provided by the journal.
Collections
Scholars@Duke
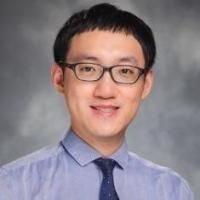
Yang Sheng
My research interest focuses on machine learning and AI application in radiation oncology treatment planning, including prostate cancer, head-and-neck cancer and pancreatic cancer etc.
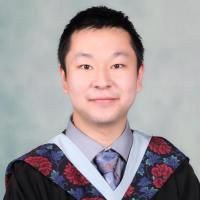
Chunhao Wang
- Deep learning methods for image-based radiotherapy outcome prediction and assessment
- Machine learning in outcome modelling
- Automation in radiotherapy planning and delivery

Qingrong Wu
Unless otherwise indicated, scholarly articles published by Duke faculty members are made available here with a CC-BY-NC (Creative Commons Attribution Non-Commercial) license, as enabled by the Duke Open Access Policy. If you wish to use the materials in ways not already permitted under CC-BY-NC, please consult the copyright owner. Other materials are made available here through the author’s grant of a non-exclusive license to make their work openly accessible.