Identifying vulnerable brain networks associated with Alzheimer's disease risk.
Date
2023-04
Journal Title
Journal ISSN
Volume Title
Repository Usage Stats
views
downloads
Citation Stats
Attention Stats
Abstract
The selective vulnerability of brain networks in individuals at risk for Alzheimer's disease (AD) may help differentiate pathological from normal aging at asymptomatic stages, allowing the implementation of more effective interventions. We used a sample of 72 people across the age span, enriched for the APOE4 genotype to reveal vulnerable networks associated with a composite AD risk factor including age, genotype, and sex. Sparse canonical correlation analysis (CCA) revealed a high weight associated with genotype, and subgraphs involving the cuneus, temporal, cingulate cortices, and cerebellum. Adding cognitive metrics to the risk factor revealed the highest cumulative degree of connectivity for the pericalcarine cortex, insula, banks of the superior sulcus, and the cerebellum. To enable scaling up our approach, we extended tensor network principal component analysis, introducing CCA components. We developed sparse regression predictive models with errors of 17% for genotype, 24% for family risk factor for AD, and 5 years for age. Age prediction in groups including cognitively impaired subjects revealed regions not found using only normal subjects, i.e. middle and transverse temporal, paracentral and superior banks of temporal sulcus, as well as the amygdala and parahippocampal gyrus. These modeling approaches represent stepping stones towards single subject prediction.
Type
Department
Description
Provenance
Subjects
Citation
Permalink
Published Version (Please cite this version)
Publication Info
Mahzarnia, Ali, Jacques A Stout, Robert J Anderson, Hae Sol Moon, Zay Yar Han, Kate Beck, Jeffrey N Browndyke, David B Dunson, et al. (2023). Identifying vulnerable brain networks associated with Alzheimer's disease risk. Cerebral cortex (New York, N.Y. : 1991), 33(9). pp. 5307–5322. 10.1093/cercor/bhac419 Retrieved from https://hdl.handle.net/10161/32034.
This is constructed from limited available data and may be imprecise. To cite this article, please review & use the official citation provided by the journal.
Collections
Scholars@Duke

Hae Sol Moon
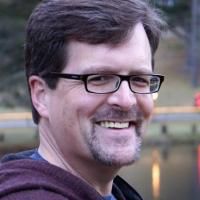
Jeffrey Nicholas Browndyke
Dr. Browndyke is an Associate Professor of Behavioral Health & Neurosciences in the Department of Psychiatry & Behavioral Sciences. He has a secondary appointment as Assistant Professor of Cardiovascular & Thoracic Surgery.
Dr. Browndyke's research interests involve the use of advanced neurocognitive and neuroimaging techniques for perioperative contributions to delirium and later dementia risk, monitoring of late-life neuropathological disease progression, and intervention/treatment outcomes. His research also involves novel telehealth methods for remote neurocognitive evaluation and implementation of non-invasive neuromodulatory techniques to assist in postoperative recovery and dementia risk reduction.
Dr. Browndyke's clinical expertise is focused upon geriatric neuropsychology with an emphasis in the assessment, diagnosis, and treatment of dementia and related disorders in adults and US veteran patient populations.
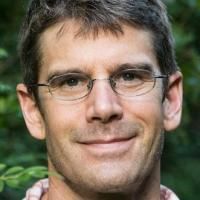
David B. Dunson
My research focuses on developing new tools for probabilistic learning from complex data - methods development is directly motivated by challenging applications in ecology/biodiversity, neuroscience, environmental health, criminal justice/fairness, and more. We seek to develop new modeling frameworks, algorithms and corresponding code that can be used routinely by scientists and decision makers. We are also interested in new inference framework and in studying theoretical properties of methods we develop.
Some highlight application areas:
(1) Modeling of biological communities and biodiversity - we are considering global data on fungi, insects, birds and animals including DNA sequences, images, audio, etc. Data contain large numbers of species unknown to science and we would like to learn about these new species, community network structure, and the impact of environmental change and climate.
(2) Brain connectomics - based on high resolution imaging data of the human brain, we are seeking to developing new statistical and machine learning models for relating brain networks to human traits and diseases.
(3) Environmental health & mixtures - we are building tools for relating chemical and other exposures (air pollution etc) to human health outcomes, accounting for spatial dependence in both exposures and disease. This includes an emphasis on infectious disease modeling, such as COVID-19.
Some statistical areas that play a prominent role in our methods development include models for low-dimensional structure in data (latent factors, clustering, geometric and manifold learning), flexible/nonparametric models (neural networks, Gaussian/spatial processes, other stochastic processes), Bayesian inference frameworks, efficient sampling and analytic approximation algorithms, and models for "object data" (trees, networks, images, spatial processes, etc).
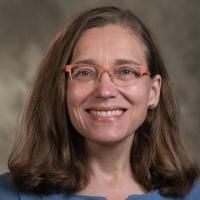
Kim G Johnson
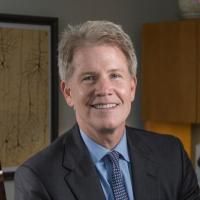
Richard J O'Brien

Alexandra Badea
I have a joint appointment in Radiology and Neurology and my research focuses on neurological conditions like Alzheimer’s disease. I work on imaging and analysis to provide a comprehensive characterization of the brain. MRI is particularly suitable for brain imaging, and diffusion tensor imaging is an important tool for studying brain microstructure, and the connectivity amongst gray matter regions.
I am interested in image segmentation, morphometry and shape analysis, as well as in integrating information from MRI with genetics, and behavior. Our approaches target: 1) phenotyping the neuroanatomy using imaging; 2) uncovering the link between structural and functional changes, the genetic bases, and environmental factors. I am interested in generating methods and tools for comprehensive phenotyping.
We use high-performance cluster computing to accelerate our image analysis. We use compressed sensing image reconstruction, and process large image arrays using deformable registration, perform segmentation based on multiple image contrasts including diffusion tensor imaging, as well as voxel, and graph analysis for connectomics.
At BIAC my efforts focus on developing multivariate biomarkers and identifying vulnerable networks based on genetic risk for Alzheimer's disease.
My enthusiasm comes from the possibility to extend from single to integrative multivariate and network based analyses to obtain a comprehensive picture of normal development and aging, stages of disease, and the effects of treatments. I am working on multivariate image analysis and predictive modeling approaches to help better understand early biomarkers for human disease indirectly through mouse models, as well as directly in human studies.
I am dedicated to supporting an increase in female presence in STEM fields, and love working with students. The Bass Connections teams involve undergraduate students in research, providing them the opportunity to do independent research studies and get involved with the community. These students have for example takes classes such as:
BME 394: Projects in Biomedical Engineering (GE)
BME 493: Projects in Biomedical Engineering (GE)
ECE 899: Special Readings in Electrical Engineering
NEUROSCI 493: Research Independent Study 1
Unless otherwise indicated, scholarly articles published by Duke faculty members are made available here with a CC-BY-NC (Creative Commons Attribution Non-Commercial) license, as enabled by the Duke Open Access Policy. If you wish to use the materials in ways not already permitted under CC-BY-NC, please consult the copyright owner. Other materials are made available here through the author’s grant of a non-exclusive license to make their work openly accessible.