A neural network-based method for spectral distortion correction in photon counting x-ray CT.
Date
2016-08
Journal Title
Journal ISSN
Volume Title
Repository Usage Stats
views
downloads
Citation Stats
Attention Stats
Abstract
Spectral CT using a photon counting x-ray detector (PCXD) shows great potential for measuring material composition based on energy dependent x-ray attenuation. Spectral CT is especially suited for imaging with K-edge contrast agents to address the otherwise limited contrast in soft tissues. We have developed a micro-CT system based on a PCXD. This system enables both 4 energy bins acquisition, as well as full-spectrum mode in which the energy thresholds of the PCXD are swept to sample the full energy spectrum for each detector element and projection angle. Measurements provided by the PCXD, however, are distorted due to undesirable physical effects in the detector and can be very noisy due to photon starvation in narrow energy bins. To address spectral distortions, we propose and demonstrate a novel artificial neural network (ANN)-based spectral distortion correction mechanism, which learns to undo the distortion in spectral CT, resulting in improved material decomposition accuracy. To address noise, post-reconstruction denoising based on bilateral filtration, which jointly enforces intensity gradient sparsity between spectral samples, is used to further improve the robustness of ANN training and material decomposition accuracy. Our ANN-based distortion correction method is calibrated using 3D-printed phantoms and a model of our spectral CT system. To enable realistic simulations and validation of our method, we first modeled the spectral distortions using experimental data acquired from (109)Cd and (133)Ba radioactive sources measured with our PCXD. Next, we trained an ANN to learn the relationship between the distorted spectral CT projections and the ideal, distortion-free projections in a calibration step. This required knowledge of the ground truth, distortion-free spectral CT projections, which were obtained by simulating a spectral CT scan of the digital version of a 3D-printed phantom. Once the training was completed, the trained ANN was used to perform distortion correction on any subsequent scans of the same system with the same parameters. We used joint bilateral filtration to perform noise reduction by jointly enforcing intensity gradient sparsity between the reconstructed images for each energy bin. Following reconstruction and denoising, the CT data was spectrally decomposed using the photoelectric effect, Compton scattering, and a K-edge material (i.e. iodine). The ANN-based distortion correction approach was tested using both simulations and experimental data acquired in phantoms and a mouse with our PCXD-based micro-CT system for 4 bins and full-spectrum acquisition modes. The iodine detectability and decomposition accuracy were assessed using the contrast-to-noise ratio and relative error in iodine concentration estimation metrics in images with and without distortion correction. In simulation, the material decomposition accuracy in the reconstructed data was vastly improved following distortion correction and denoising, with 50% and 20% reductions in material concentration measurement error in full-spectrum and 4 energy bins cases, respectively. Overall, experimental data confirms that full-spectrum mode provides superior results to 4-energy mode when the distortion corrections are applied. The material decomposition accuracy in the reconstructed data was vastly improved following distortion correction and denoising, with as much as a 41% reduction in material concentration measurement error for full-spectrum mode, while also bringing the iodine detectability to 4-6 mg ml(-1). Distortion correction also improved the 4 bins mode data, but to a lesser extent. The results demonstrate the experimental feasibility and potential advantages of ANN-based distortion correction and joint bilateral filtration-based denoising for accurate K-edge imaging with a PCXD. Given the computational efficiency with which the ANN can be applied to projection data, the proposed scheme can be readily integrated into existing CT reconstruction pipelines.
Type
Department
Description
Provenance
Citation
Permalink
Published Version (Please cite this version)
Publication Info
Touch, Mengheng, Darin P Clark, William Barber and Cristian T Badea (2016). A neural network-based method for spectral distortion correction in photon counting x-ray CT. Physics in medicine and biology, 61(16). 10.1088/0031-9155/61/16/6132 Retrieved from https://hdl.handle.net/10161/16500.
This is constructed from limited available data and may be imprecise. To cite this article, please review & use the official citation provided by the journal.
Collections
Scholars@Duke
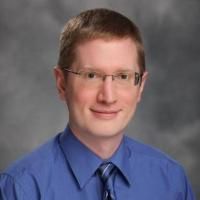
Darin Clark
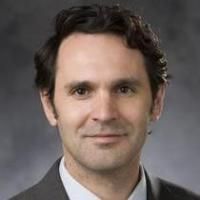
Cristian Tudorel Badea
- Our lab's research focus lies primarily in developing novel quantitative imaging systems, reconstruction algorithms and analysis methods. My major expertise is in preclinical CT.
- Currently, we are particularly interested in developing novel strategies for spectral CT imaging using nanoparticle-based contrast agents for theranostics (i.e. therapy and diagnostics).
- We are also engaged in developing new approaches for multidimensional CT image reconstruction suitable to address difficult undersampling cases in cardiac and spectral CT (dual energy and photon counting) using compressed sensing and/or deep learning.
- We are involved in co-clinical cancer trials and I have served as the Principal Investigator on the U24 Duke Preclinical Research Resources for Quantitative Imaging Biomarkers part of the NCI Co-Clinical Imaging Research Resources Program network (CIRP).
Unless otherwise indicated, scholarly articles published by Duke faculty members are made available here with a CC-BY-NC (Creative Commons Attribution Non-Commercial) license, as enabled by the Duke Open Access Policy. If you wish to use the materials in ways not already permitted under CC-BY-NC, please consult the copyright owner. Other materials are made available here through the author’s grant of a non-exclusive license to make their work openly accessible.